Written by: Sumit Shee
July 16, 2023
Overview:
As the world gets more digitized, the importance of data has grown in a variety of fields, including cybersecurity and artificial intelligence (AI). However, the rise of data poisoning has created a new threat scenario with far-reaching consequences. Injecting dangerous or deceptive information into datasets is known as data poisoning, and it can have a substantial influence on the effectiveness and dependability of cybersecurity systems and AI algorithms. In this essay, we will look at the effects of data poisoning and how it affects the security and integrity of both cyber systems and artificial intelligence.
Cybersecurity Data Poisoning: Cybersecurity systems rely largely on data to identify and mitigate threats. Adversaries, on the other hand, can introduce flaws and elude discovery if they modify the data used to train these systems. Attackers can exploit weaknesses, circumvent security measures, and gain unauthorized access to systems by purposely contaminating training data with harmful samples or subtly modifying benign data.
One such example is spam filter poisoning. Attackers can add false positives and negatives into the training data, increasing the rate of false negatives (genuine emails tagged as spam) and false positives (spam emails evading detection). This manipulation can have serious repercussions, such as phishing attempts bypassing security defences, compromising sensitive data, or harming key infrastructure.
Data Poisoning in AI: The impact of data poisoning extends beyond cybersecurity. To learn and generate correct predictions, AI systems, such as machine learning and deep learning models, rely largely on massive amounts of high-quality data. Adversaries can alter AI models’ behaviour by manipulating training data, resulting in inaccurate or biased outputs.
For example, in autonomous driving, an attacker may introduce tiny adjustments to the training data needed to teach an AI system how to recognise traffic signs, causing the AI to misclassify stop signs or recognize non-existent objects. This might have severe implications on the road, endangering the safety of both drivers and pedestrians.
Mitigation Strategies: Dealing with the consequences of data poisoning necessitates a proactive and multifaceted strategy. Here are some important mitigation strategies:
Robust Data Validation: During the data collecting phase, thorough data validation techniques assist identify and filter out possibly contaminated data.
Adversarial Training: Including adversarial instances and scenarios during the training process might improve the model’s resistance to attacks and capacity to recognize and discard poisoned data.
Continuously monitoring AI models and their input data can assist spot any abnormalities or deviations from predicted behaviour, allowing for prompt intervention and retraining if necessary.
Data Source Diversity: By diversifying the data sources used to train AI models, the danger of poisoning from a single corrupted source is reduced.
Conclusion:
Data poisoning is a major threat to both cybersecurity and AI systems, eroding their dependability and effectiveness while potentially jeopardizing security and safety. As technology advances, organizations and researchers must remain watchful and implement strong measures to detect, prevent, and reduce the consequences of data poisoning. We can improve the resilience of our cyber and AI systems by recognizing this threat and deploying appropriate defences, providing a safer and more secure digital future.
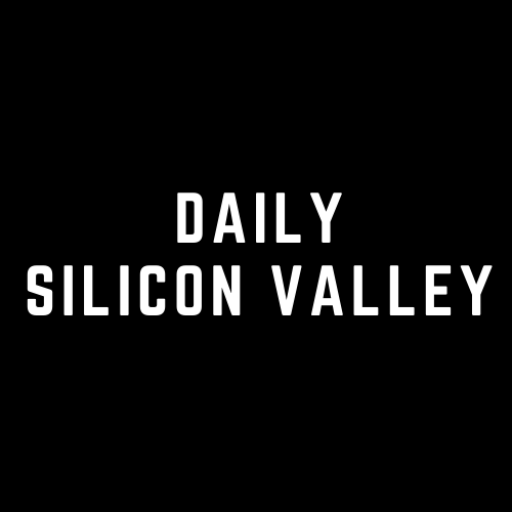
Daily magazine for entrepreneurs and business owners