To the Google Foundation and Working Groups on Analytics
Dr. Jonathan Kenigson, FRSA*
The scientific revolution of the 17th century occasioned a profound and indissoluble union among mathematics, mechanics, and the physical sciences. Within 100 years or so after Copernicus and Kepler, and after millennia of ostensible detachment, it became nigh-on impossible to conceive of science without the structure and rigor of mathematics. Modern philosophers and logicians disagree as to whether mathematics and physics can – even in principle – be disabused from their byzantine connections. After Einstein, Newton, Kelvin, and Maxwell, one would be required to resort to the most abstruse domains of philosophical discourse to cleave mathematics from physics and propose to find any intelligible remainder.
In a similar manner, contemporary data science and statistics – though allied disciplines – are not interchangeable, and knowledge of one domain does not confer automatic facility in the other (Hong et al., 2020). Statistics is comparably indispensable to data science as geometry and analysis are indispensable to the physical sciences. More generally, mathematics – though arguably not a science – efficiently facilitates the development, communication, formalization, and evaluation of scientific thought. Mathematicians and statisticians are not scientists, but rather logicians whose methods of inquiry are intuitive and (at least inwardly) artistic. Pure mathematicians ponder and agonize over hastily scrawled diagrams which are erased as thoroughly and rapidly as possible, lest anyone should be scandalized by the notion that a picture-in-itself could ever prove anything. Modern mathematics and statistics are thus predominantly anti-visual domains: These disciplines loathe the image; they persist in, and subsist upon, the ministry of the syllogism and the quantified type.
There are no illuminated manuscripts in reasonably contemporary mathematical discourse. As it is practiced by mathematicians, statistics is bounded inexorably by the expansive theories of probability and calculus. Logical metatheories, epsilons, and limitless excursions into nether-worldly abstraction conspire to render pictures alongside professional proofs as philistine and unprofessional artefacts. Mathematical statistics is a discipline in which ironclad proof for assertions is more important than the assertions themselves. This is the ponderous, austere, and monochrome road of Kolmogorov, Fomin, Pearson, and the grand scions of the discipline. Whereas the poets of human languages have robust commerce in visual metaphor and allusion, the mathematician merely exploits diagrams for their expediency, neglecting (or even resisting) the persuasive power of the visual aesthetic in-toto. It is precisely this laconic paradigm and its attendant austerities that the modern data scientist rejects – both in favor of the empirical and in ever-mindful pursuit of the practical and profitable.
Data scientists are, first and foremost, scientific artists – or, rather more properly, facilitators of machines’ artistic paideia. Unlike previous epochs, humans and machines collaborate in their common sciences, but it is the humans who teach art and the machines that produce and exegete the same. As we have discussed, statistical theory is pure mathematics, and the methods of statistics – although occasionally computational – do not rely at all upon computing for demonstration (Kim & Escobedo-Land, 2015). Data science could not be more different in its approach to images, icons, and visualizations: It unifies statistics, data analysis, numerics, computational geometry, applied computer science, and graphical design to draw actionable insights from large and complex data sets (Varshney et al., 2017). This science is, as its name suggests, a true and empirical enterprise: It encompasses the testing and rejection of hypotheses won by observation and intuition rather than pure deduction and systematic proof (Galeano & Peña, 2019). Arguments are sculpted, painted, and rendered lively by machines’ boundless capacity to find order in the chaos of petascale data.
Data science has the potential to drive rapid development in domains previously restricted by the practical difficulties posed by the visualization of massive, disordered, and chaotic datasets. Such contributions are characterized by the restoration of the primacy of the visual – as opposed to the merely logical – facets of practical-logical discourse. As disparate cases-in-point, we may briefly consider manifold forms of healthcare analytics and climate science. In healthcare, data science will facilitate improved patient care via the generation and evaluation of immersive graphical interfaces to model disease progression; likely and possible courses of treatment for complex diseases; bespoke drug design; radiological imaging analysis via automated prediction algorithms; and highly personalized medical interventions afforded by computational genetics and environmental analysis (Dunn & Bourne, 2017; Stoicescu et al., 2021; Tomy et al., 2021). In climate studies, data science will furnish profoundly interactive visualizations regarding the progression and remediation of anthropogenic climate change by organizations, governments, non-governmental organizations, and households (Majda et al., 2009; Majda et al. 2010; Mulvaney & Druschke, 2017). Similar methods will permit targeted digital education on the dangers of human-induced climate change (Chu & Yang, 2020; Duram, 2021; Mohapatra et al., 2022; Nation & Feldman, 2022; Petrescu-Mag et al., 2022).
If mathematics is the “language” of science – then statistics must be the dialect most closely suited to the science of data. Data science, however, would be unrecognizable without the rich and multifarious diagrams inaugurated by the capabilities of artificial intelligence, neural networks, pattern recognition algorithms, and massively parallel computing. Concise and compelling graphics explain – albeit to some partial degree – the extent to which data science can rapidly expand entire domains of knowledge that statistics only enriched via tortuous and plodding inquiry.
Respectfully,
J. Kenigson
Nashville, TN, USA
References:
Agbo, S., Ifeoluwa, Y., & Eric, A. (2022). Forecasting premium motor spirit (pms) and energy commodities prices using machine learning techniques: a review. Umyu Scientifica, 1(1), 194-203. https://doi.org/10.56919/usci.1122.025
Aler, R., Martín, R., Valls, J., & Galván, I. (2015). A study of machine learning techniques for daily solar energy forecasting using numerical weather models., 269-278. https://doi.org/10.1007/978-3-319-10422-5_29
Anuar, A., Hussain, N., Masrom, S., Mohd, T., Ahmad, S., & Ahmad, N. (2023). Reverse migration factor in machine learning models. International Journal of Academic Research in Business and Social Sciences, 13(2). https://doi.org/10.6007/ijarbss/v13-i2/16282
Baumer, B. (2015). A data science course for undergraduates: thinking with data. The American Statistician, 69(4), 334-342. https://doi.org/10.1080/00031305.2015.1081105
Belapurkar, S. (2019). Machine learning approach for identification of diseases through gene mapping. International Journal for Research in Applied Science and Engineering Technology, 7(5), 650-652. https://doi.org/10.22214/ijraset.2019.5112
Bolhuis, M. and Rayner, B. (2020). The more the merrier? a machine learning algorithm for optimal pooling of panel data. IMF Working Paper, 20(44). https://doi.org/10.5089/9781513529974.001
Buontempo, C., Burgess, S., Dee, D., Pinty, B., Thépaut, J., Rixen, M., … & Marcilla, J. (2022). The Copernicus climate change service: climate science in action. Bulletin of the American Meteorological Society, 103(12), E2669-E2687. https://doi.org/10.1175/bams-d-21-0315.1
Cheong, T., Shi, X., Li, Y., & Sun, Y. (2022). Editorial: application of big data, deep learning, machine learning, and other advanced analytical techniques in environmental economics and policy. Frontiers in Environmental Science, 10. https://doi.org/10.3389/fenvs.2022.953659
Chu, H. and Yang, J. (2020). Their economy and our health: communicating climate change to the divided American public. International Journal of Environmental Research and Public Health, 17(21), 7718. https://doi.org/10.3390/ijerph17217718
Dartevelle, O., Altomonte, S., Masy, G., Mlecnik, E., & Moeseke, G. (2022). Indoor summer thermal comfort in a changing climate: the case of a nearly zero energy house in Wallonia (Belgium). Energies, 15(7), 2410. https://doi.org/10.3390/en15072410
Defontaine, T., Ricci, S., Lapeyre, C., Marchandise, A., & Pape, E. (2023). Flood forecasting with machine learning in a scarce data layout. IOP Conference Series Earth and Environmental Science, 1136(1), 012020. https://doi.org/10.1088/1755-1315/1136/1/012020
Dunn, M. and Bourne, P. (2017). Building the biomedical data science workforce. PLOS Biology, 15(7), e2003082. https://doi.org/10.1371/journal.pbio.2003082
Duram, L. (2021). Teaching a social science course on climate change: suggestions for active learning. Bulletin of the American Meteorological Society, 102(8), E1494-E1498. https://doi.org/10.1175/bams-d-21-0035.1
Ferreira, W., Grout, I., & Silva, A. (2020). Forecasting energy time-series data using a fuzzy Artmap neural network. https://doi.org/10.1109/icpei49860.2020.9431435
Fu, T., Zhou, H., Ma, X., Hou, Z., & Wu, D. (2022). Predicting peak day and peak hour of electricity demand with ensemble machine learning. Frontiers in Energy Research, 10. https://doi.org/10.3389/fenrg.2022.944804
Galeano, P. and Peña, D. (2019). Data science, big data and statistics. Test, 28(2), 289-329. https://doi.org/10.1007/s11749-019-00651-9
Gokul, K., Sundararajan, D., & Paul, P. (2019). Big data management, data science and data analytics: what is it and where— an educational in Indian perspective. International Journal of Innovative Technology and Exploring Engineering, 8(12), 1231-1237. https://doi.org/10.35940/ijitee.l3978.1081219
Hong, L., Moen, W., Yu, X., & Chen, J. (2020). The disciplinary research landscape of data science reflected in data science journals. Information Discovery and Delivery, 49(4), 287-297. https://doi.org/10.1108/idd-06-2020-0071
Hota, S., Jena, S., Gupta, B., & Mishra, D. (2020). An empirical comparative analysis of nav forecasting using machine learning techniques., 565-572. https://doi.org/10.1007/978-981-15-6202-0_58
Letchumanan, K. and Naveen, P. (2022). Machine learning regression models to predict particulate matter (pm2.5)., 458-468. https://doi.org/10.2991/978-94-6463-094-7_36
Liu, J., Yuan, X., Zeng, J., Jiao, Y., Li, Y., Zhong, L., … & Yao, L. (2022). Ensemble streamflow forecasting over a cascade reservoir catchment with integrated hydrometeorological modeling and machine learning. Hydrology and Earth System Sciences, 26(2), 265-278. https://doi.org/10.5194/hess-26-265-2022
Lohia, K., Garg, S., Shrivastava, N., & Panigrahi, B. (2015). Comparative study of power forecasting methods for wind farms. https://doi.org/10.1109/iccpct.2015.7159429
Maehashi, K. and Shintani, M. (2020). Macroeconomic forecasting using factor models and machine learning: an application to Japan. Journal of the Japanese and International Economies, 58, 101104. https://doi.org/10.1016/j.jjie.2020.101104
Majda, A., Abramov, R., & Gershgorin, B. (2009). High skill in low-frequency climate response through fluctuation dissipation theorems despite structural instability. Proceedings of the National Academy of Sciences, 107(2), 581-586. https://doi.org/10.1073/pnas.0912997107
Majda, A., Gershgorin, B., & Yuan, Y. (2010). Low-frequency climate response and fluctuation–dissipation theorems: theory and practice. Journal of the Atmospheric Sciences, 67(4), 1186-1201. https://doi.org/10.1175/2009jas3264.1
Masum, S., Chiverton, J., Liu, Y., & Vuksanović, B. (2019). Investigation of machine learning techniques in forecasting of blood pressure time series data., 269-282. https://doi.org/10.1007/978-3-030-34885-4_21
Mohapatra, S., Mallik, K., & Satapathy, M. (2022). Education for sustainable development: a study on the attitude and concerns on climate change issues among elementary school teachers of Odisha, India. Asian Journal of Education and Social Studies, 37-52. https://doi.org/10.9734/ajess/2022/v30i430732
Mulvaney, K. and Druschke, C. (2017). Using diverse expertise to advance climate change fisheries science. Ocean & Coastal Management, 149, 175-185. https://doi.org/10.1016/j.ocecoaman.2017.10.006
Nation, M. and Feldman, A. (2022). Climate change and political controversy in the science classroom. Science & Education, 31(6), 1567-1583. https://doi.org/10.1007/s11191-022-00330-6
Petrescu-Mag, R., Burny, P., Banatean-Dunea, I., & Petrescu, D. (2022). How climate change science is reflected in people’s minds. a cross-country study on people’s perceptions of climate change. International Journal of Environmental Research and Public Health, 19(7), 4280. https://doi.org/10.3390/ijerph19074280
Schweizer, S., Thompson, J., Teel, T., & Bruyere, B. (2009). Strategies for communicating about climate change impacts on public lands. Science Communication, 31(2), 266-274. https://doi.org/10.1177/1075547009352971
Sharma, S., Ghimire, G., & Siddique, R. (2022). Machine learning for postprocessing ensemble streamflow forecasts. Journal of Hydroinformatics, 25(1), 126-139. https://doi.org/10.2166/hydro.2022.114
Tamminen, S., Siirtola, P., Chandra, G., Veijola, R., … & Röning, J. (2021). Clinflow – an interactive application for clinical data mining. https://doi.org/10.3233/shti210162
Sujjaviriyasup, T. and Pitiruek, K. (2013). Agricultural product forecasting using a machine learning approach. International Journal of Mathematical Analysis, 7, 1869-1875. https://doi.org/10.12988/ijma.2013.35113
Sujjaviriyasup, T. and Pitiruek, K. (2013). Hybrid Arima-support vector machine model for agricultural production planning. Applied Mathematical Sciences, 7, 2833-2840. https://doi.org/10.12988/ams.2013.13251
Tomy, L., Chesneau, C., & Madhav, A. (2021). Statistical techniques for environmental sciences: a review. Mathematical and Computational Applications, 26(4), 74. https://doi.org/10.3390/mca26040074
Varshney, M., Garg, S., & Rajpoot, J. (2017). A study on issues, challenges and application in data science. International Journal of Trend in Scientific Research and Development, Volume-1(Issue-5), 526-533. https://doi.org/10.31142/ijtsrd2340
Wang, J. (2017). Research on machine learning and its algorithms. Destech Transactions on Computer Science and Engineering, (cii). https://doi.org/10.12783/dtcse/cii2017/17243
Wilms, H., Cupelli, M., & Monti, A. (2018). Combining auto-regression with exogenous variables in sequence-to-sequence recurrent neural networks for short-term load forecasting. https://doi.org/10.1109/indin.2018.8471953
Zhou, C., Li, H., Chen, Y., Xia, J., & Zhang, P. (2022). A station-data-based model residual machine learning method for fine-grained meteorological grid prediction. Applied Mathematics and Mechanics, 43(2), 155-166. https://doi.org/10.1007/s10483-022-2822-9
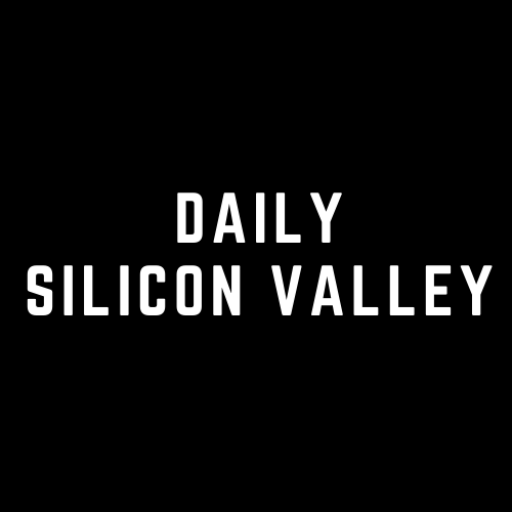
Daily magazine for entrepreneurs and business owners