written by Arsen ILHAN
Introduction:
Artificial Intelligence (AI) integration in businesses demands a strategic approach aligned with unique organizational goals. This article will be discussing the key strategies for effective AI implementation in businesses, encompassing objectives, data-centricity, technology selection, ethical considerations, continuous improvement, employee collaboration, customer-centric applications, risk management, and iterative evaluation. The integration of Artificial Intelligence (AI) within businesses has become pivotal in driving innovation, optimizing operations, and achieving competitive advantage. However, effective AI adoption requires a nuanced and strategic approach tailored to each company’s unique objectives and capacities.
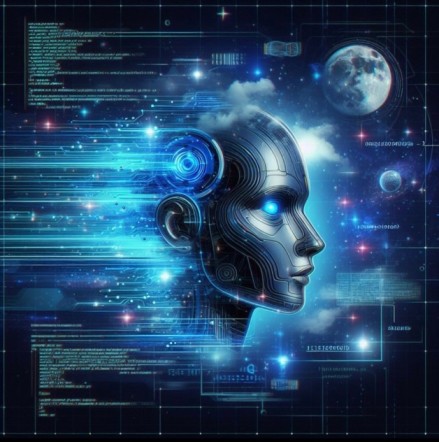
AI strategies
The journey towards AI integration commences with a meticulous identification of specific business challenges or opportunities ripe for AI intervention. Whether it’s streamlining supply chains, automating routine tasks, or personalizing customer interactions, establishing precise objectives anchors AI initiatives towards tangible outcomes.
AI thrives on data. At the core of AI prowess lies data. Accumulating, organizing, and utilizing quality data is fundamental. The strategic accumulation, organization, and utilization of high quality data serve as the lifeblood of AI algorithms. Businesses must invest in data collection methods, storage infrastructure, and data analytics to ensure they have the right information to feed AI algorithms. Google’s search engine algorithms rely on vast amounts of data collected from user searches, website crawls, and user behavior. They continually accumulate and analyze this data to enhance search relevance, understand user intent, and deliver more accurate search results. Facebook gathers diverse user interaction data, including likes, shares, comments, and post interactions. This data is instrumental in training AI algorithms to personalize user feeds, target ads, and moderate content, improving user engagement and platform safety.
There’s a multitude of AI technologies available, from machine learning and natural language processing to computer vision. Understanding the strengths and limitations of each technology helps in selecting the most suitable tools for specific business needs.Google developed TensorFlow, an open-source machine learning framework. It offers a diverse range of tools and libraries suitable for various machine learning tasks. Microsoft’s Azure AI Services offer a suite of AI APIs for vision, speech, language, and decision-making. These APIs allow developers to integrate AI capabilities into applications, empowering businesses with AI-driven functionalities.
Using AI ethically is crucial for responsible business. It goes beyond just following rules; it’s a commitment to societal values and principles. Prioritizing ethics in AI implementation encompasses a multifaceted approach that resonates deeply in today’s interconnected world. Being clear about how AI makes decisions is key for trust between businesses and stakeholders. It’s not just about revealing the inner workings; it’s about helping people understand why AI reaches specific conclusions. For instance, in lending institutions, transparent AI algorithms can explain why a loan application was accepted or rejected, instilling trust and understanding among applicants. Safeguarding data privacy is not merely a legal requirement but a moral obligation. Ensuring that personal information remains shielded within AI systems forms the bedrock of user trust. A stark example is the fallout from data breaches in social media platforms, underscoring the repercussions when privacy is compromised and the urgent need for robust data protection measures in AI. Mitigating biases within AI systems is pivotal to uphold fairness and equity. Biases, often inadvertently embedded in AI algorithms, can perpetuate discrimination. A classic example is biased facial recognition technology that exhibits racial or gender biases, emphasizing the urgency to proactively detect, acknowledge, and rectify biases within AI systems. The importance of ethical AI extends far beyond compliance; it embodies a commitment to fostering a responsible technological ecosystem. When businesses prioritize ethical use of AI, they not only mitigate risks of legal ramifications but also nurture trust, promote inclusivity, and pave the way for a more ethically conscious technological landscape. This approach not only safeguards businesses from potential backlash but also nurtures enduring relationships with stakeholders based on trust, integrity, and societal values.
The convergence of AI literacy among employees and the harmonious collaboration between AI systems and human expertise is a pivotal catalyst in amplifying productivity, fostering innovation, and augmenting problem-solving capacities within enterprises. Empowering personnel with a profound understanding of AI intricacies not only cultivates a workforce proficient in harnessing the transformative potential of AI but also lays the groundwork for a seamless fusion of AI-driven technologies with human intellect.This synergy between AI literacy and collaborative interaction between systems fortified by human acumen opens gateways to revolutionizing customer experiences. AI, adept at personalization, predictive analytics, and the seamless integration of chatbots, emerges as the linchpin in tailoring bespoke solutions precisely attuned to meet diverse customer needs. The finesse of this tailored approach not only garners loyalty but also nurtures a deeper sense of customer satisfaction, forging enduring relationships between businesses and their clientele. Combining human know-how with AI boosts customer experiences, making them more engaging and satisfying.
Indeed, the bedrock of sustainable success in AI implementation lies not in a singular deployment but in the orchestration of a continuous cycle of learning and refinement. This iterative journey begins with the establishment of meticulous metrics, finely tuned to gauge the multifaceted effectiveness of AI initiatives. These metrics serve as compass points, guiding the meticulous analysis of performance indicators at regular intervals. Through this diligent analysis, insights blossom, offering a panoramic view of AI’s impact and revealing nuances vital for strategic evolution. This process transcends mere evaluation; it encapsulates the dynamic essence of AI adaptation, where feedback becomes the nourishment fueling the refinement engine. The assimilation of feedback channels into AI models metamorphoses them into living entities, attuned not just to current needs but primed for the landscape of evolving business demands. This cyclical approach acknowledges that AI, akin to a maestro refining its symphony, requires an unending quest for enhancement. Continuous learning isn’t just a virtue; it’s the heartbeat that propels AI initiatives forward, ensuring that each iteration, each refinement, ushers in a new crescendo of efficacy and relevance, perpetually aligning with the evolving tapestry of business landscapes.
In the tapestry of continuous learning and refinement within AI implementation, the threads of risk management and scalability interweave, fortifying the fabric of sustainable success. Understanding the potential risks inherent in AI implementation is akin to wielding a compass amidst uncharted waters. It involves not only identifying security vulnerabilities but also anticipating the contours of unexpected outcomes that might lurk within the labyrinth of evolving algorithms. Yet, this vigilance extends beyond risk mitigation; it converges with the pursuit of scalability. AI’s power to smoothly adjust to changing business needs is crucial. It helps navigate shifts in the business landscape effectively. Scalability becomes the cornerstone that bridges the present with the future, ensuring that AI initiatives are not just solutions for today but flexible, agile entities poised to meet the challenges of tomorrows yet unknown. The synergy between risk management and scalability within the realm of continuous learning denotes a strategic balancing act. It’s the art of fostering an environment where calculated risks, meticulously managed, coexist with the fluidity of scalable solutions, all under the constant gaze of assessment, adaptation, and evolution. This harmonious interplay secures the path forward, empowering AI initiatives to navigate through uncertainties and chart trajectories of sustainable growth and resilience.
In conclusion, successful integration of AI into business operations demands a strategic and holistic approach. By defining clear objectives, leveraging data effectively, ensuring ethical practices, and fostering a culture of continuous improvement, businesses can unlock the true potential of AI and drive sustainable growth in today’s competitive landscape.
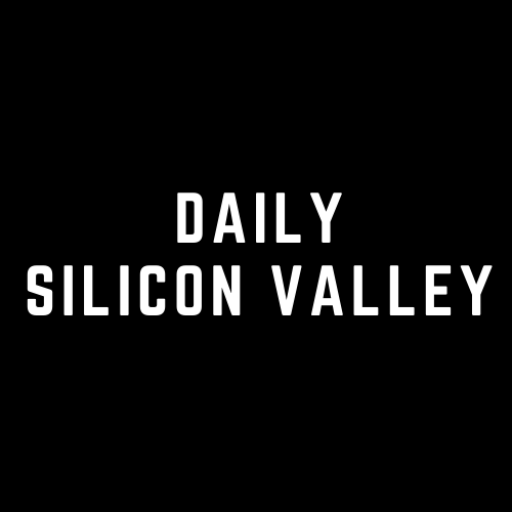
Daily magazine for entrepreneurs and business owners